Data Analysis and Venture Capital
The world of venture capital is dominated by the likes of John Doerr, Tom Perkins, Bruce Dunlevie, and other great names who built the world in which we live now. If you read any of their memoirs – I had the pleasure of finishing Valley Boy: The Education of Tom Perkins on a recent trip to visit Limited Partners in LatAM, you get a sense for their ability to discern talent, capability and market potential of their investments. Take a read through eBoys: The First Inside Account of Venture Capitalists At Work, and you are greeted by fascinating blow-by-blow accounts of the agonizing decisions these men labored over in making their investment decisions. And through it all, you realize that historically this world, and the world of investing at large, has been dominated by the man or woman on the scene, making the best determination he (or she) can in the moment and with the thought processes available to them and their team.
However, in this day and age, we have access to more data than has been aggregated in the history of mankind. If you consider the impact of data on different industries, you get a sense for how this has markedly transformed human decision making. Think of insurance – a business we know well – and the how the ability to underwrite risk has steadily transformed the risk for the insurer and re-insurers and changed the consumer experience. Or in healthcare – imagine the benefits to researchers and doctors who are able to better parse data to find previously unknown options for treatment, etc. Data analysis has thoroughly infiltrated the hedge fund world, and also the world of Wall Street. There is no investment house now that relies exclusively on human intuition – many of the major hedge funds actually market their capability to use data analysis to find opportunities. I recall at my previous firm hearing a presentation from a hedge fund manager who explained that they were able to use satellite imagery to count the number of cars per day in parking lots of major retailers as a way of building more sophisticated models for estimating growth and investment opportunity.
So why not venture capital? Well, first of all, VC has historically been both an opaque business (from the perspective of “what are VCs investing in, and how’s it doing, and what’s their performance”, but also because the world of start-ups has historically been informal, handled with private transactions, and without the benefit of a public listing (like the stock market) to allow aggregation of data over time. Do we know the Price to Sales ratios for the world of private start-ups? Likely not.
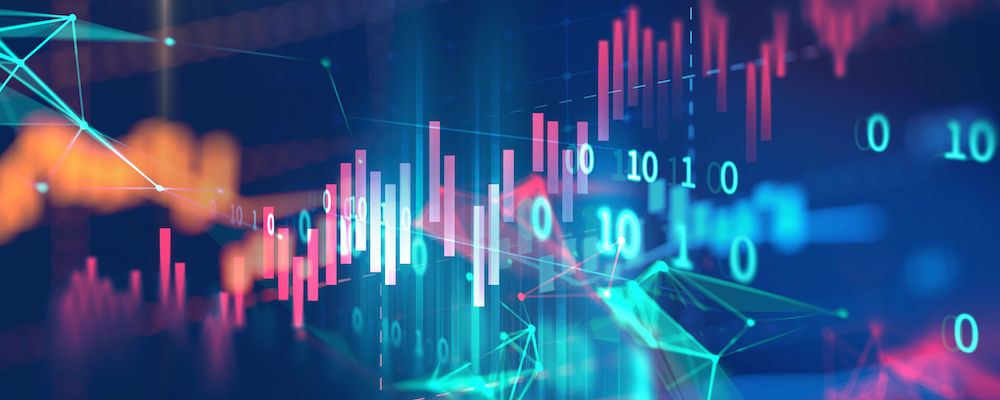
But this has begun to change, and the opportunities for data-enhanced analysis are becoming quite intriguing. Here’s a fantastic interview with Veronica Wu, the Managing Partner of Hone Capital, where she describes the way they have been able to utilize machine learning and data to better analyze opportunities. Consider the unprecedented opportunity in the private start-up world offered by platforms such as Angellist, which aggregate almost every start-up imaginable and provide the data that can inform decision making. One of the more interesting quotes, I thought, from Veronica’s article was that seed rounds that raised only $0.5mm were much less likely to advance to a Series A, while those that raised at least $1.5mm were much more likely to advance. This doesn’t mean they end up being a success, of course, but we consider not raising your Series A quantifies as a failure. I will leave it to you to contemplate the reasons why this happens. Or as Veronica also states – companies with founders from two different universities are much more likely to “advance” than two founders from the same university. As we used to say in my equity research days – there must be an economic rationale behind a correlation, or it wasn’t necessarily a legitimate conclusion.
In any event, with the amount of data now being offered to venture capitalists, we believe data analysis will only become more important as an aid to human decision making. Because at the end of the day, human analysis and judgement is still very important. I really believe that AI and ML, and the ability to crunch large amounts of numbers and come to conclusions, will always (perhaps always is a strong word, but for the very long and foreseeable future) be a major aid and complement to human decision making. Most importantly – to check biases.
I intend to write another post on this topic alone (human biases in venture capital) but consider these three very human capabilities. Intuition, pattern matching and judgment. The first and the third are truly in the domain of humans (with major aid from machines), and pattern matching can arguably be done even better by a machine. However, here’s the rub – all three can lead an investor astray. If nothing else, data analysis and informed decision-making serves as an effective check on human biases. It can confirm or deny your intuition, it can identify patterns which the human is then able to draw conclusions from, and can be a major aid in wisdom and judgment.
For example – take any decision about humans (aka founders). Does university degree (or the type of degree) make a difference? Impossible to say with say 10 samples. But maybe there’s some statistical correlation when you have more than 10,000 samples? Or more? How about number of direct reports to the CEO / Founder / CTO (you name it)? Does the intended use for the raise matter in the success? Does the availability of tech talent matter, or do great companies attract great talent? On this vein, how about geographical location? Does a company founded in Silicon Valley have better odds of success than a company springing up in Charlotte, NC? My inclination is yes – but that means absolutely nothing without some deeper understanding of the data. But even then, data can still lead you astray, and so a human judge is always necessary to analyze and interpret the data. In the end, my own conclusion is that venture capitalists should aspire to gather and utilize more data in their decision making. But in the end, human wisdom, judgment and connection still matter a great deal, and one should never surrender their autonomy to the tyranny of data.